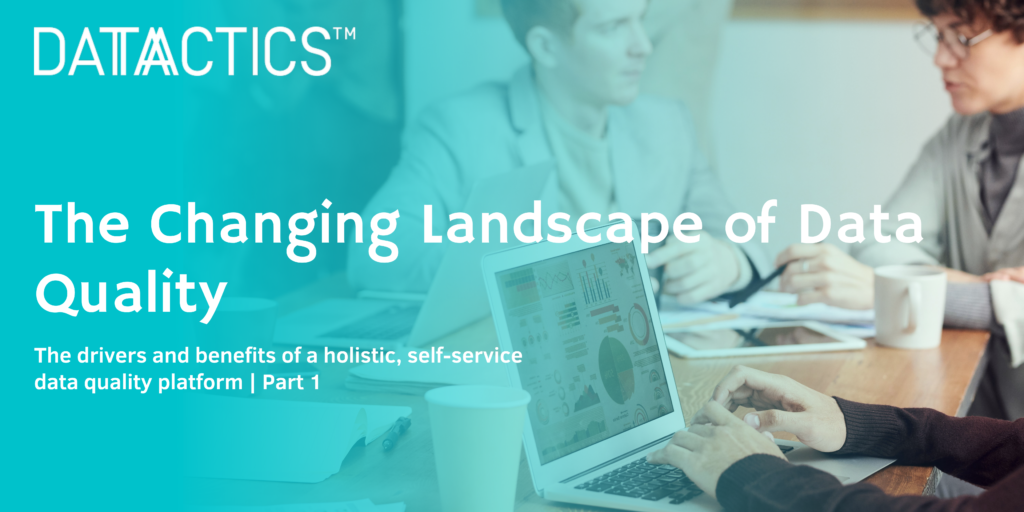
The drivers and benefits of a holistic, self-service data quality platform | Part 1
Change
There has been increasing demand for higher and higher data quality in recent years – highly regulated sectors, such as banking have had a tsunami of financial regulations such as BCBS239, MiFID, FATCA, and many more stipulating or implying exacting standards for data and data processes. Meanwhile, there is a growing trend for more and more firms to become more Data and Analytics (D&A) driven, taking inspiration from Google & Facebook, to monetize their data assets.
This increased focus on D&A has been accelerated by easier and lower-cost access to artificial intelligence (AI), machine learning (ML), and business intelligence (BI) visualization technologies. However, in the now-waning hype of these technologies comes the pragmatic realization that unless there is a foundation of good quality reliable data, insights derived from AI and analytics may not be actionable. With AI and ML becoming more of a commodity, and a level playing field, the differentiator is in the data and the quality of the data.
“Unless there is a foundation of good quality reliable data, insights derived from AI and analytics may not be actionable”
Problems
As the urgency for regulatory compliance or competitive advantage escalates, so too does the urgency for high data quality. A significant obstacle to quickly achieve high data quality is the variety of disciplines required to measure data quality, enrich data and fix data. By its nature, digital data, especially big data can require significant technical skills to manipulate and for this reason, was once the sole responsibility of IT functions within an organization. However, maintaining data also requires significant domain knowledge about the content of the data, and this domain knowledge resides with the subject matter experts (SMEs) who use the data, rather than a central IT function. Furthermore, each data set will have its own SMEs with special domain knowledge required to maintain the data, and a rapidly growing and changing number of data sets. If a central IT department is to maintain the quality of data correctly it must therefore liaise with an increasingly large number of data owners and SMEs in order to correctly implement DQ controls and remediation required. These demands create a huge drain on IT resources and a slow-moving backlog of data quality change requests within IT that simply can’t keep up.
Given the explosion in data volumes, this model clearly won’t scale and so there is now a growing trend to move data quality operations away from central IT and back into the hands of data owners. While this move can greatly accelerate data quality and data onboarding processes, it can be difficult and expensive for data owners and SMEs to meet the technical challenges of maintaining and onboarding data. Furthermore, unless there is common governance around data quality across all data domains there stands the risk of a ‘wild west’ scenario, where every department manages data quality differently with different processes and technology.
Opportunity
The application of data governance policies and the creation of an accountable Chief Data Officer (CDO) goes a long way to mitigate against the ‘wild west’ scenario. Data quality standards such as the Enterprise Data Management Council’s (EDMC) Data Capability Assessment Model (DCAM)1 provide opportunities to establish consistency in data quality measurement across the board.
The drive to capitalize on data assets for competitive advantage has had the result that the CDO function is quickly moving from an operational cost centre towards a product-centric profit centre. A recent publication by Gartner (30th July 2019) 2 describes three generations of CDO: “CDO 1.0” focused on data management; “CDO 2.0” embraced analytics; “CDO 3.0” assisted digital transformation, and Gartner now predicts a fourth, “CDO 4.0” focused on monetizing data-oriented products. Gartner’s research suggests that to enable this evolution, companies should strive to develop data and analytics platforms that scale across the entire company and this implies data quality platforms that scale too.
To have further conversations about the drivers and benefits of a Self-Service Data Quality platform, book a quick call with Kieran Seaward.
And for more from Datactics, find us on Linkedin, Twitter, or Facebook.