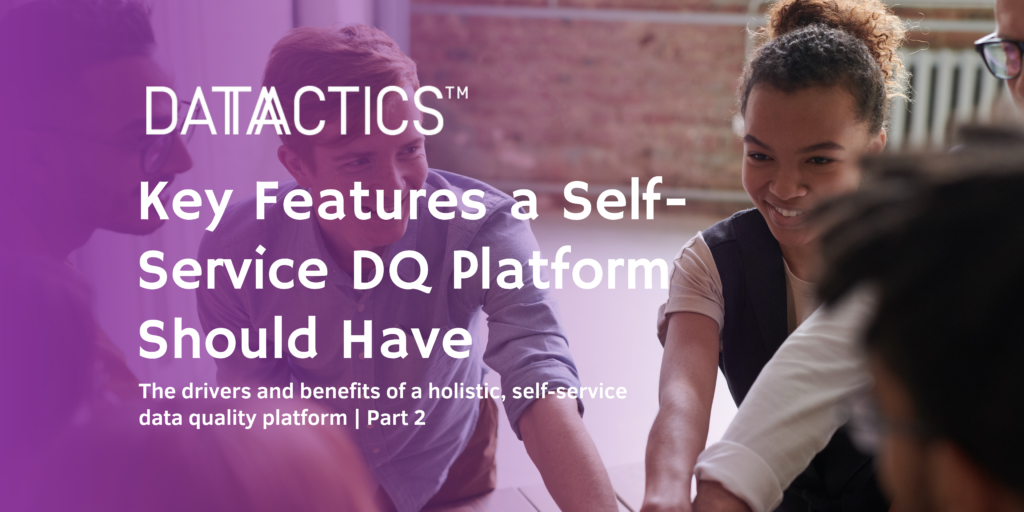
The drivers and benefits of a holistic, self-service data quality platform | Part 2
To enable the evolution towards actionable insight from data, D&A platforms and processes must evolve too. At the core of this evolution is the establishment of ‘self-service’ data quality – whereby data owners and SMEs have ready access to robust tools and processes, to measure and maintain data quality themselves, in accordance with data governance
policies. From a business perspective such a self-service data quality platform must be:
❖ Powerful enough to enable business users and SMEs to perform complex data operations
without highly skilled technical assistance from IT
❖ Transparent, accountable and consistent enough to comply with firm wide data governance
policies
❖ Agile enough to quickly onboard new data sets and changing data quality demands of end
consumers such as AI and Machine learning algorithms
❖ Flexible and open so it integrates easily with existing data infrastructure investment without
requiring changes to architecture or strategy
❖ Advanced to make pragmatic use of AI and machine learning to minimize manual
intervention
This goes way beyond the scope of most stand-alone data prep tools and ‘home grown’ solutions that are often used as a tactical one-off measure for a particular data problem. Furthermore, for the self-service data quality platform to truly enable actionable data across the enterprise, it will need to provide some key technical functionality built-in:
• Transparent & Continuous Data Quality Measurement
Not only should it be easy for business users and SMEs to implement large numbers of data domain specific data quality rules, but also those rules should be simple to audit, and easily explainable, so that ‘DQ breaks’ can be easily explored and the root cause of the break established.
In addition to data around the actual breaks, a DQ platform should be able to produce DQ dashboards enabling drill-down from high level statistics down to actual failing data points and publish high level statistics into data governance systems.
• Powerful Data Matching – Entity Resolution for Single View and Data Enrichment
Finding hidden value in data or complying with regulation very often involves joining together several disparate data sets. For example, enhancing a Legal Entity Master Database with an LEI, screening customer accounts against sanctions and PEP lists for KYC, creating a single view of client from multiple data silos for GDPR or FSCS compliance. This goes further than simple deduplication of records or SQL joins – most data sets are messy and don’t have unique identifiers and so fuzzy matching of numerous string fields must be implemented to join one data set with another. Furthermore, efficient clustering algorithms are required to sniff out similar records from other disparate data sets in order to provide a single consolidated view across all silos.
• Integrated Data Remediation Incorporating Machine Learning
It’s not enough just to flag up broken data, you also need a process and technology for fixing the breaks. Data quality platforms should have this built in so that after data quality measurement, broken data can be quarantined, data owners alerted and breaks automatically assigned to the relevant SMEs for remediation Interestingly, the manual remediation process lends itself very well to machine learning. The process of manually remediating data captures domain specific knowledge about the data – information that can be readily used by machine learning algorithms to streamline the resolution of similar breaks in the future and thus greatly reduce the overall time and effort spent on manual remediation.
“The process of manually remediating data captures domain specific knowledge about the data – information that can be readily used by machine learning algorithms to streamline the resolution of similar breaks in the future”
• Data Access Controls Across Teams and Datasets
Almost any medium to large sized organization will have various forms of sensitive data, and policies for sharing that data within the organization e.g. ‘Chinese walls’ between one department and another. In order to enable integration across teams and disparate silos of data, granular access controls are required – especially inside the data remediation technology where sensitive data may be displayed to users. Data access permissions should be set automatically where possible (e.g. inheriting Active Directory attributes) and enforced when displaying data, for example by row- and field-level access control, and using data masking or obfuscation where appropriate.
- Audit Trails, Assigning and Tracking Performance
Providing business users with tools to fix data could cause additional headaches when it comes to being able to understand who did what, when, why and whether or not it was the right thing to do. It stands to reason, therefore, that any remediation tool should have builtin capability to do just that with the associated performance of data break remediation
measured, tracked and managed.
- AI Ready
There’s no doubt that one of the biggest drivers of data quality is AI. AI data scientists can spend up to 80% of their time just preparing input data for machine learning algorithms, which is a huge waste of their expertise. A self-service data quality platform can address many of the data quality issues by providing ready access to tools and processes that can ensure a base level of quality and identify anomalies in data that may skew machine learning models. Furthermore the same self-service data quality tools can assist data scientists to generate metadata that can be used to inform machine learning models – such ‘Feature Engineering’ can be of real value when the data set is largely textual as it can generate numerical indicators which are more readily consumed by ML algorithms.
“AI data scientists can spend up to 80% of their time just preparing input data for machine learning algorithms, which is a huge waste of their expertise”
To have further conversations about the drivers and benefits of a Self-Service Data Quality platform, please book a quick call with Kieran Seaward.
And for more from Datactics, find us on Linkedin, Twitter, or Facebook.