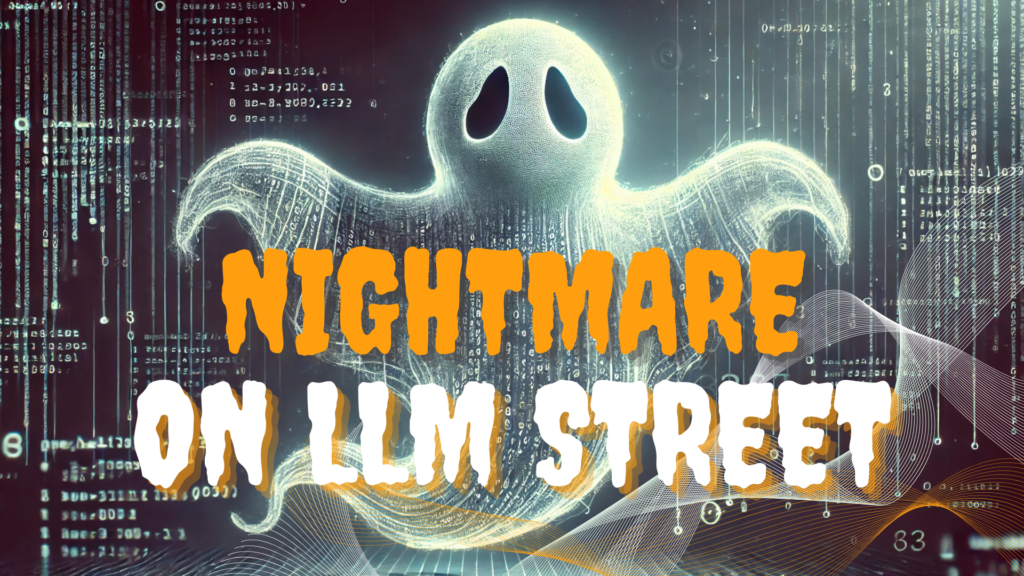
It’s October, the Northern Hemisphere nights are drawing in, and for many it’s time when things take a scarier turn. But for public sector leaders exploring AI, that fright need not apply to your data. It definitely shouldn’t be something that haunts your digital transformation dreams.
With a reported £800m budget unveiled by the previous government to address ‘digital and AI’, UK public sector departments are keen to be the first to explore the sizeable benefits that AI and automation offer. The change of government in July 2024 has done nothing to indicate that this drive has lessened in any way; in fact, the Labour manifesto included the commitment to a “single unique identifier” to “better support children and families”[1].
While we await the first Budget of this Labour government, it’s beyond doubt that there is an urgent need to tackle this task amid a cost-of-living crisis, with economies still trying to recover from the economic shock of COVID and deal with energy price hikes amid several sizeable international conflicts.
However, like Hollywood’s best Halloween villains, old systems, disconnected data, and a lack of standardisation are looming large in the background.
Acting First and Thinking Later
It’s completely understandable that the pressures would lead us to this point. Societal expectations from the emergence of ChatGPT, among others, have only fanned the flames, swelling the sense that technology should just ‘work’ and leading to an overinflated belief in what is possible.
Recently, LinkedIn attracted some consternation[i][2] by automatically including members’ data in its AI models without seeking express consent first. For whatever reason, the idea that people would just accept this change was overlooked. It took the UK’s Information Commissioner’s Office, the ICO, to intervene for the change to be withdrawn – in the UK, at least.
A dose of reality is the order of the day. Government systems are lacking integrated data, and clear consent frameworks of the type that LinkedIn actually possesses seldom exist in one consistent way. Already lacking funds, the public sector needs to act carefully, and mindfully, to prevent their AI experiments (which is, after all, what they are) from leading to inaccuracies and wider distrust from the general public.
Streamlining Data and Consent in One Single View
One solution is for Government departments to form one, holistic set of consents concerning use of data for AI, especially Large Language Models and Generative AI – similar to communication consents under the General Data Protection Regulation, GDPR.
The adoption of a flexible consent management policy, one which can be updated and maintained for future developments and tied to an interoperable, standardised single view of citizen (SCV), will serve to support the clear, safe development of AI models into the future. The risks of building models now, on shakier foundations, will only serve to erode public faith. The evidence of the COVID-era exam grades fiasco[3] demonstrates the risk that these models present to real human lives.
Of course, it’s not easy to do. Many legacy systems contain names, addresses and other citizen data in a variety of formats. This makes it difficult to be sure that when more than one dataset includes a particular name, that name actually refers to the same individual. Traditional solutions to this problem use anything from direct matching technology to the truly awful exercise of humans manually reviewing tens of thousands of records in spreadsheets. This is one recurring nightmare that society really does need to stop having.
Taking Refuge in Safer Models
Intelligent data matching uses a variety of matching algorithms and well-established machine learning techniques to reconcile data held in old systems, new ones, documents, even voice notes. Such approaches could help the public sector to streamline their SCV processes, managing consents more effectively. The ability to understand who has opted in, marrying opt-ins and opt-outs to demographic data is critical. This approach will help model creators to interpret the inherent bias in the models built on those consenting to take part, to understand how reflective of society the predictive models are likely to be – including whether or not it is actually safe to use the model at all.
It’s probable that this transparency in process could also lead to greater trust in the general public to take part in data sharing in this way. In the LinkedIn example, the news that data was being used without explicit consent, raced around like wildfire on the platform itself. This sort of outcome cannot be what LinkedIn anticipated, which in and of itself is a concern about the mindset of the model creators.
It Doesn’t Have to Be a Nightmare
It’s a spooky enough season without adding more fear to the bonfire; certainly, this article isn’t intended as a reprimand. The desire to save time and money to deliver better services to a country’s citizens is a major part of many a civil servant’s professional drive. And AI and automation offer so many opportunities for much better outcomes! For just one example, NHS England’s AI tool already uses image recognition to detect heart disease up to 30 times faster than a human[4] . Mid and South Essex (MSE) NHS Foundation used a predictive analytical machine learning model called Deep Medical to reduce the rate at which patients either didn’t attend appointments or cancelled with short notice (referred to as Did Not Attend, or DNA). Its pilot project identified which patients were more likely to fall into the DNA category, developed personalised reminder schedules, and through identifying frail patients who were less likely to attend an appointment, highlighted them to relevant clinical teams.[5]
The time for taking action is now. Public sector organisations, government departments and agencies should focus on the need to develop systems that will preserve and maintain trust in the AI-led future. This blog has shown that better is possible, through a dedicated desire to align citizen data and their consents to contact. In a society where people have trust and transparency in the ways that their data will be used to train AI, the risk of nightmare scenarios can be averted and we’ll all sleep better at night.
[1] https://www.ropesgray.com/en/insights/viewpoints/102jc9k/labour-victory-the-implications-for-data-protection-ai-and-digital-regulation-i
[2] https://etedge-insights.com/in-focus/trending/linkedin-faces-backlash-for-using-user-data-in-ai-training-without-consent/
[3] https://www.ncbi.nlm.nih.gov/pmc/articles/PMC7894241/#:~:text=COVID%2D19%20prompted%20the%20UK,teacher%20assessed%20grades%20and%20standardisation.
[4] https://www.healthcareitnews.com/news/emea/nhs-rolls-out-ai-tool-which-detects-heart-disease-20-seconds
[5] https://www.nhsconfed.org/publications/ai-healthcare