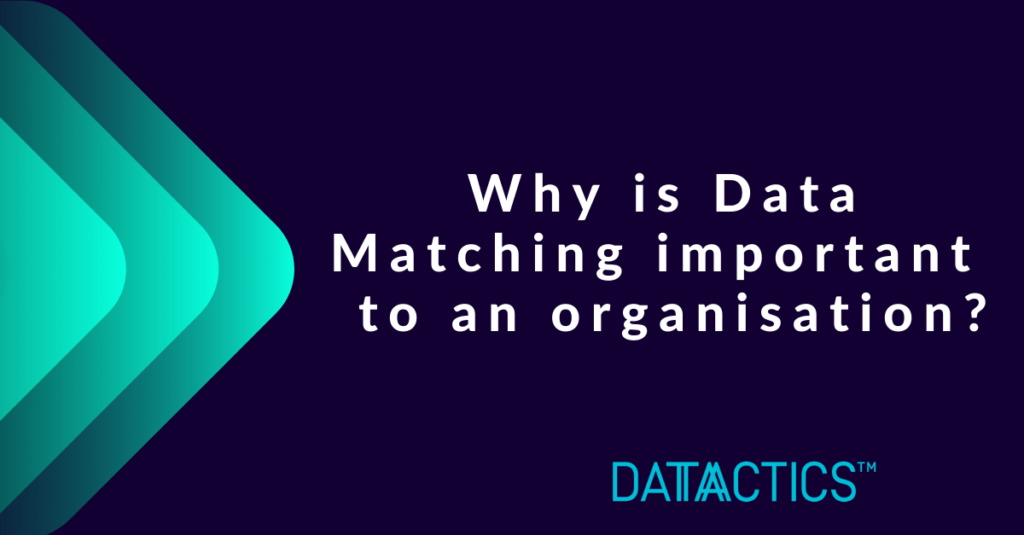
Data Matching has a huge part to play in every element of data management, whether in maintaining data standards for regulatory compliance or in perfecting data for digital transformation and actionable business intelligence.
In this blog, Jamie Gordon delves into the wider implications of data matching and the need for different match strategies.
Data Matching is a fundamental element of master data management, data governance and data quality. It is one of the key requirements of a technology-driven solution to allow for data to be compared, patterns to be identified and discrepancies to be flagged. Data matching also helps to enable greater standards of accuracy whilst keeping irrelevant information to a minimum.
For example, a retail bank might use data matching to understand customer habits in order to build better cross-selling relationships with them. In a government setting, a department could use data matching technology to create a single view of citizens to support vulnerable people. On the compliance side, financial institutions risk losing vast sums of money through fines by having large volumes of messy data.
The truth is that nowadays, every organisation is built on its data assets – whether it realises it or not. The problem that matching tries to solve is knowing that two ‘entities’ are, in fact, the same ‘entity’.
Where data matching technology helps
Data matching technology can be extremely low-latency, which means that organisations can perform millions of matches within seconds and remove manual effort which in contrast often takes days, weeks or even months. Often, companies can be unknowingly wasting resources by using out of date data. This can be remediated by using match tooling to assist with maintaining records across various databases and reducing the presence of duplicate records.
How does data matching technology work?
There are many ways to perform data matching. Frequently, the process is based on a matching algorithm or a programmed loop, where each piece of the data set is compared and matched against each piece of the other data set.
The data needs to be sorted, or blocked, into similar-sized blocks with the same attribute. These should be attributes that are unlikely to change, such as names, dates of birth, colour, or shape. Typically this data can be referred to as ‘master data.’
Then there are different match strategies to be used depending on the data being matched. Names, for example, are often matched phonetically, and very often firms need to employ translation or transliteration capabilities – where data is matched across languages – to identify matches that have been recorded in (for example) Arabic and English. Matching algorithms can also be supported by the use of AI and Machine Learning (AI/ML), to identify patterns within and across datasets which could be matches. Employing multiple match strategies is increasingly becoming the choice for leading firms who understand the importance of their data assets.
How to choose a matching strategy
IT and business leaders need to ask the question: What is the most sustainable process for matching to ensure the highest level of precision and accuracy? Being able to understand the business context, and employing data quality tools to ensure the underlying data being matched is fit for purpose, are two such building blocks of an effective match strategy decision. The appropriateness and reliability of AI/ML models, as well as the purpose of the matching exercise, are two further elements which must be borne in mind in any matching project.
To have further conversations about how Datactics is helping data leaders to match individuals and entities against combined UK, EU and US sanctions lists, please book a quick call with Kieran Seaward.
Sign up here to try out our fuzzy-matching, transliteration and phonic matching capabilities in our free-to-use Sanctions Match Engine.
And for more from Datactics, find us on Linkedin, Twitter or Facebook.