Looking for a more recent article on data governance? Head here.
A few years ago, Heineken broadcast a classic Elephant-in-the-room advert where various groups of utility construction workers all agree to carry out their work at the same time, to “save digging up the road again and causing the public more inconvenience.”
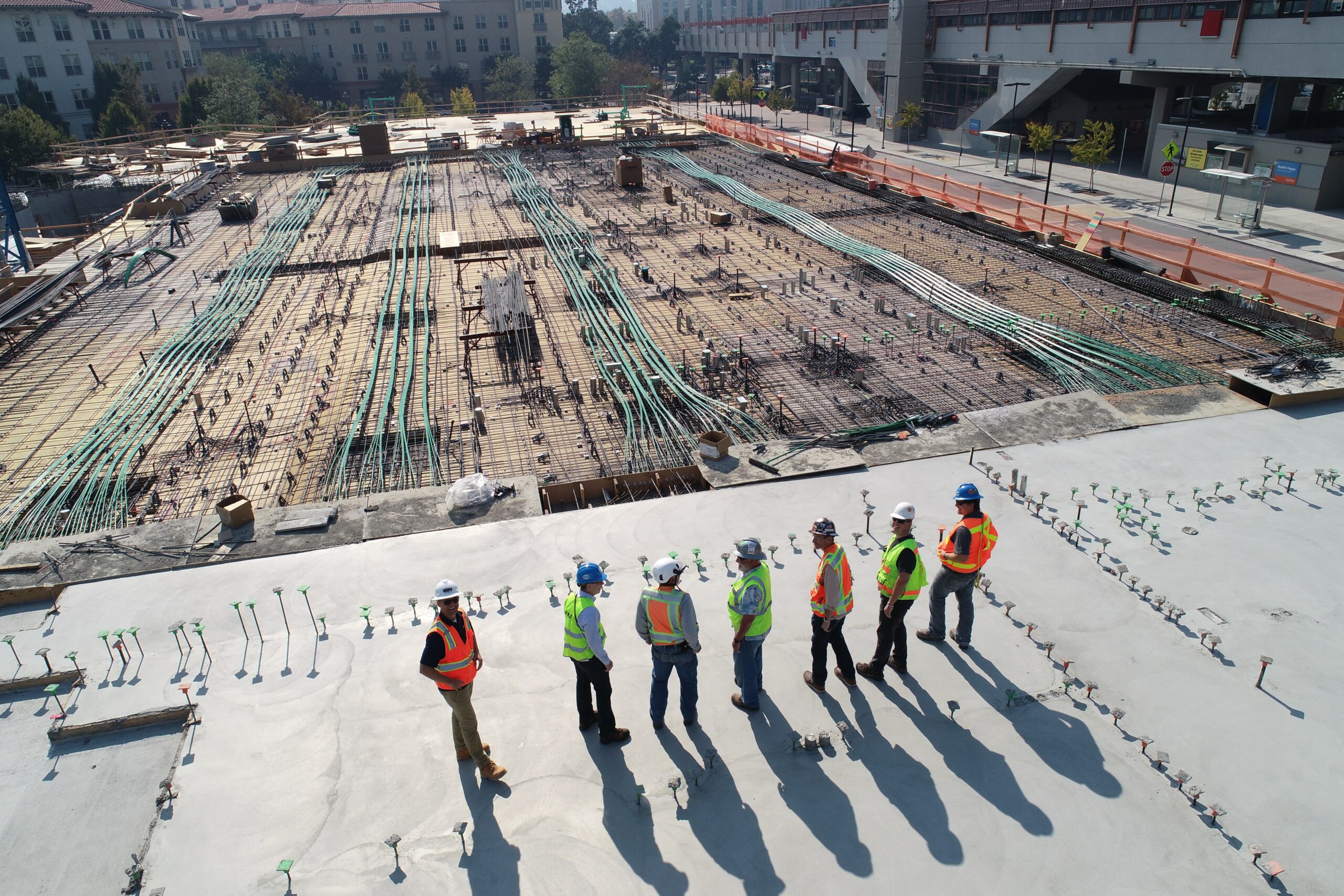
It’s probably the dream of every road-weary traveller encountering another set of roadworks on their already long journey.
Of course, it’s fine in theory, but as heads of Data, Regulatory Reporting or Analytics functions in retail banking know, rarely are their departments connected together in such a way as to make this kind of customer-satisfying, joined-up approach becomes a reality. For one thing, the goals of each unit are usually heavily focused on the core activities of the unit:- The Head of Data will prioritise things like security, consistency and protection;
- Those reporting to regulatory bodies need to meet external criteria across a wide range of data formats so as to comply and thus avoid sanction and penalty;
- Analytics teams want to answer the great question of “how do I build my business from optimising the vast array of data I am collecting?”
- Time. Just like our friends contemplating the work ahead of them in the hole in the road, there’s usually only time to do what we need to do rather than plan for what we want to do. Regulatory compliance dates hurtle forward at breakneck speed, they frequently feature projects leveraging manual workarounds and then have a correspondingly painful transition into day-to-day operation. There’s always a reason why now isn’t the time.
- Ownership. Gather the departmental heads around the conference table and it’s not long before someone is either asking, or avoiding, the topic of who should be “on the hook” and responsible for the data at hand. Delivering any kind of change or improvement will be made far more complex if this issue isn’t sorted out.
- Priority. As stated earlier, different teams have different priorities and this can hamper efforts to make even the smallest of changes. Budgetary constraints can also have an impact on where priorities are placed.
What this means for The Data Governance Model
The starting point of the Data Governance model needs to be high quality, core personal information connected to the customer, rather than any regulation or the monetising of analytics. These should always be secondary or tertiary outcomes arising from a central process of truly knowing things like: do I really know who my customers are? Do I know where they live and work, and for how long they’ve been there? Do I know their credit and business history? Can I contact them in an emergency? In the advert, it’s only because the teams all converge at the same point that they end up saving time, effort and customer inconvenience. They all arrived equipped and ready to act. This is the key that retail banks need to use if they want to tie up a Data Governance model that works towards continuous improvement. This means that as well as an enterprise-wide master data management programme to align data requirements for the organisation – known as “top-down” Data Governance Model – work needs to start at the opposite end – “bottom-up” – to correct and continuously improve data quality issues. To achieve this, it’s vital that individual teams have access to tools that will monitor data quality and provide specific, actionable intelligence on how to remediate records that are inconsistent, duplicated, or out of date. They need to be able to consult external record databases such as credit reference agencies or Open Data business information sources to augment records that customers either didn’t keep up to date, or were recorded incorrectly at point of capture. They need to utilise machine learning and high-quality data rules so that – in time – these records can fix themselves to a standard that then meets changing regulatory standards or lead generation models. This will release people in the organisation to do what customers want them to do best: listening to, and helping other people. Delivering this level of quality intelligence on customers will ultimately save – and make – money by:- Speeding up internal processes for on-boarding (thanks to improved AML information);
- Reducing wastage and regulatory risk when contacting customers;
- Improving the quality and reliability of analytical models;
- Making IT transformation to new platforms or systems far more accurate;
- Enabling faster, more accurate regulatory compliance (e.g. FSCS, BBSI);
- Reducing manual activity in remediation and reconciliation.