There is a lot of anticipation surrounding Artificial Intelligence (Al) and Machine Learning (ML) in the media. Alongside the anticipation is speculation – including many articles placing fear into people by inferring that AI and ML will replace our jobs and automate our entire lives!
Dr Fiona Browne, Head of AI at Datactics recently spoke at an IRMAC (Information Resource Management Association of Canada) webinar, alongside Roger Vandomme, of Neos, to unpack what AI/ML is, some of the preconceptions, and the reasons why different approaches to ML are taken…
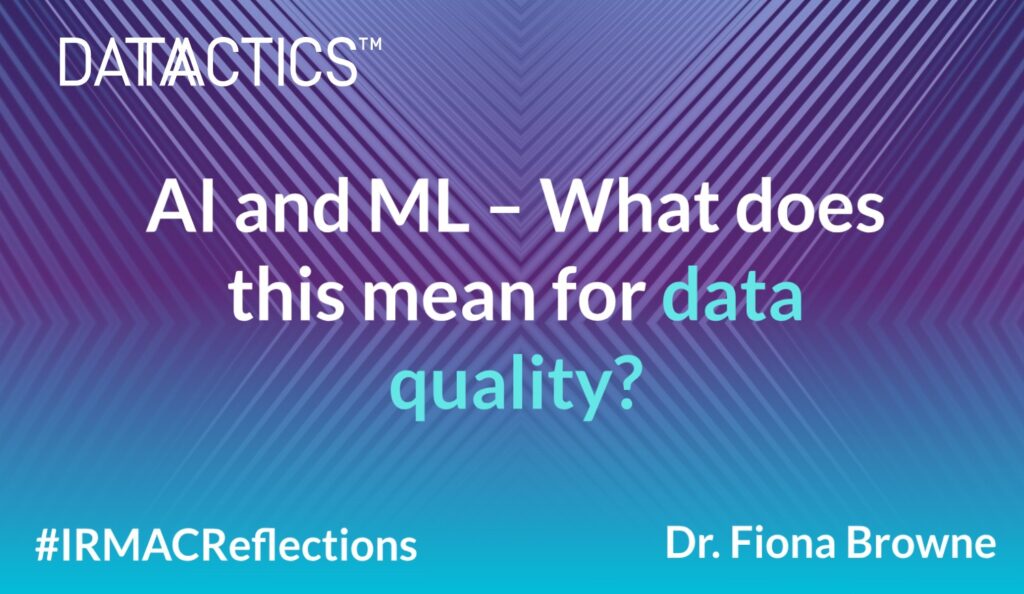
What is AI/ ML?
Dr. Browne clarified that whilst there is no official agreed-upon definition of AI, it can be depicted as the ability of a computer to perform cognitive tasks, such as voice/speech recognition, decision making, or visual perception. ML is a subset of AI, entailing different algorithms that learn from input data.
A point that Roger brought up at IRMAC was that the algorithms learn to identify patterns within the data and the used patterns enable the ability to distinguish between different outcomes, for example, the detection of a fraudulent or non-fraudulent transaction.
ML takes processes that are repetitive and automates them. At Datactics, we are exploring the usage of AI and ML in our platform capabilities – Dr Fiona Browne
What are the different approaches to ML?
Supervised, unsupervised, and reinforcement machine learning. Dr. Browne communicated that at a broad level, there are three approaches: supervised, unsupervised, and reinforcement machine learning.
In supervised ML, the model learns from a labelled training data set. For example, financial transactions would be labelled as either fraudulent or genuine fed into the ML model. The model then learns from this input and can distinguish the difference.
Where data is unlabelled, Dr. Browne explained that unsupervised ML would be more appropriate, where the model learns from unlabelled data. There is a key difference here with supervised ML in that the model would seek to uncover clusters or patterns inherent in the data to enable it to separate them out.
Finally, reinforcement machine learning involves models that continually learn and update from performing a task. For example, a computer algorithm learning how to play the game ‘Go’. This is achieved by the outputs of the model being validated and that validation being provided back to the model.
The difference between supervised learning and reinforcement learning is that in supervised learning the training data has the answer key with it, meaning the model is trained with the correct answer.
In contrast to this, in reinforcement learning, there is no answer, but the reinforcement agent selects what to do to perform the specific task.
It is important to remember that if there is no training dataset present, it is bound to learn from its experience. Often the biggest trial comes when a model is being transferred out of the training environment and into the real world.
Now that AI/ML and the different approaches have been unpacked… the next question is how does explainability fit into this? The next mini IRMAC reflection will unravel what explainability is and what the different approaches are. Stay tuned!
Fiona has written an extensive piece on AI enabled data quality, feel free to check it out here.
Click here for more by the author, or find us on Linkedin, Twitter or Facebook for the latest news.