In March 2022, Datactics took advantage of the offer to visit a local secondary school and the next generation of Data Scientists to discuss AI Ethics and Machine Learning in production. Matt Flenley shares more from the first of these two visits in his latest blog below…
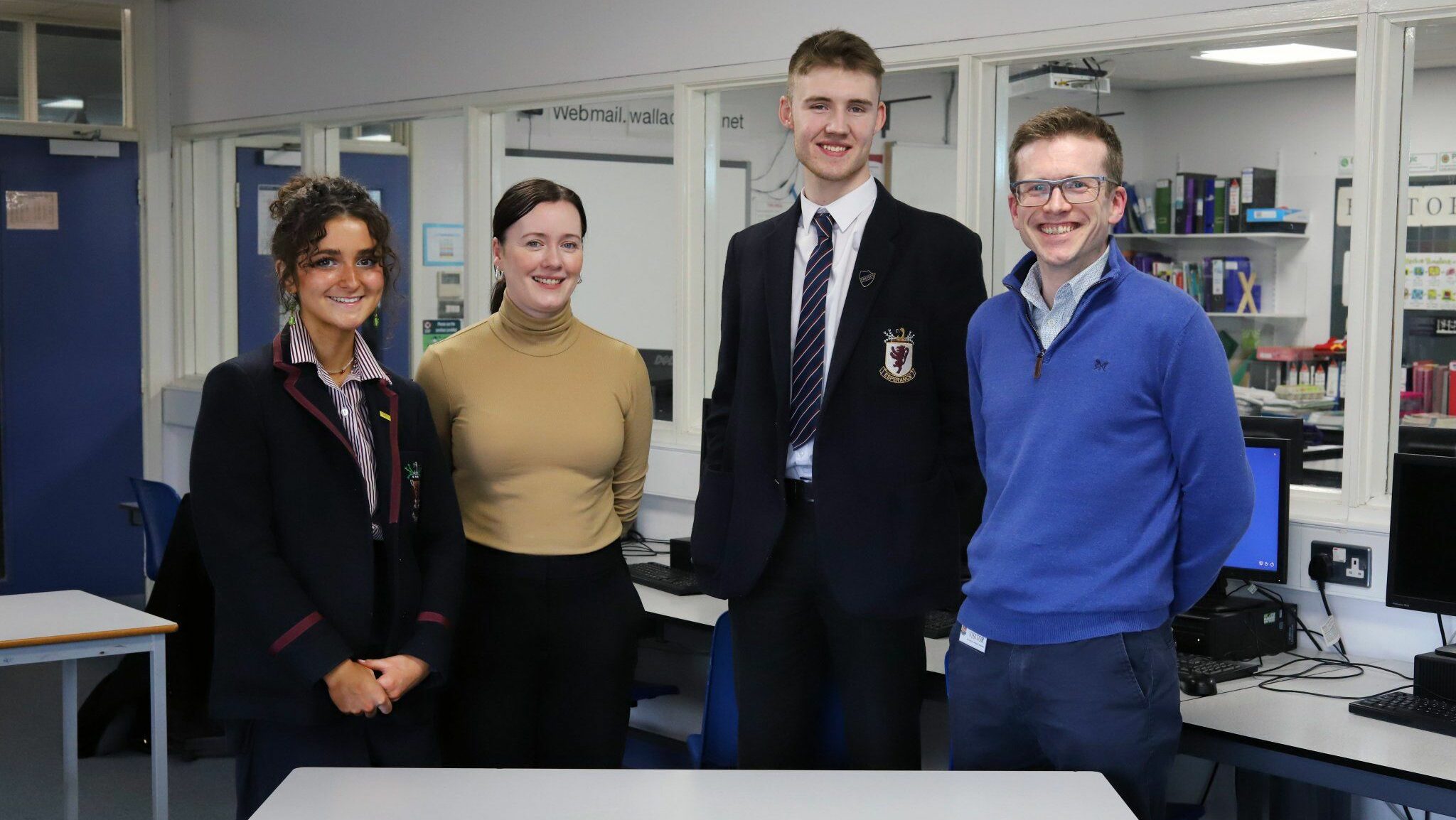
AI Ethics is often the poster child of the modern discourse on whenever the inevitable machine-led apocalypse occurs. Yet, as we look around at wars in Ukraine and Yemen, record water shortages in the developing world, and the ongoing struggle for the education of girls in Afghanistan, it becomes readily apparent that as in all things, ethics starts with humans.
This was the main thrust of the discussion with the students at Wallace High School in Lisburn, NI. As Dr Fiona Browne, Head of AI and Software Development, talked the class of second-year A-Level students through data classification for training machine learning models, the question of ‘bad actors’ came up. What if, theorised Dr Browne, people can’t be trusted to label a dataset correctly, and the machine learning model learns things that aren’t true?
At this stage, a tentative hand slowly raised in the classroom; one student confessed that, in fact, they had done exactly this in a recent dataset labelling exercise in class. It was the perfect opportunity to detail in a practical way how the human involvement in Artificial Intelligence, Machine Learning, and especially in the quality of the data underpinning both.
Humans behind the machines, and baked-in bias
As is common, the exciting part of technology is often the technology itself. What can it do? How fast can it go? Where can it take me? This applies just as much to the everyday, from home electronics through to transportation, as it does to the cutting edge of space exploration or genome mapping. However, the thought processes behind the technology, imagined up by humans, specified and scoped by humans, create the very circumstances for how those technologies will behave and interact with the world around us.
In her promotion for the book Invisible Women, the author Caroline Criado-Perez writes,
“Imagine a world where your phone is too big for your hand, where your doctor prescribes a drug that is wrong for your body, where in a car accident you are 47% more likely to be seriously injured, where every week the countless hours of work you do are not recognised or valued. If any of this sounds familiar, chances are that you’re a woman.”
Caroline Criado-Perez, Invisible Women
One example is of the comparatively high rate of anterior cruciate ligament injuries among female soccer players. While some of this can be attributed to different anatomies, it is in part caused by the lack of female-specific footwear in the sport (with most brands choosing to offer smaller sizes rather than tailored designs). Yet the anatomical design of the female knee in particular is substantially different to that of males. Has this human-led decision, to simply offer small sizes, taken into account the needs of the buyer, or the market? Has it been made from the point of view of creating a fairer society?
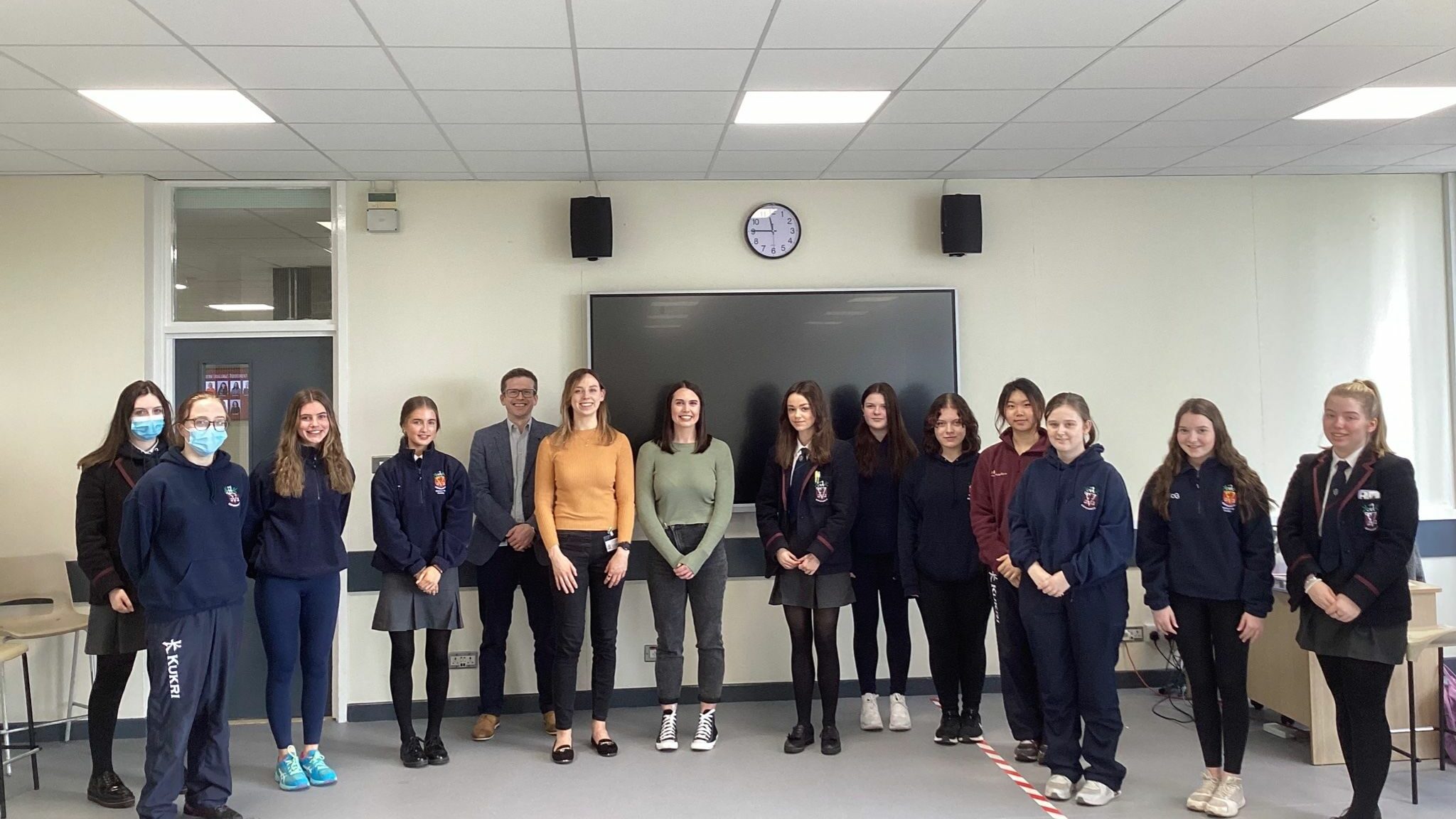
If an algorithm was therefore applied to specify a female-specific football boot from the patterns and measurements of existing footwear on the market today, would it result in a different outcome? No, of course not. It takes humans to look at the world around us, detect the risk of bias, and then do something about it.
It is the same in computing. The product, in this case the machine learning model or AI algorithm, is going to be no better than the work that has gone into defining and explaining it. A core part of this is understanding what data to use, and of what quality the data should be.
Data Quality for Machine Learning – just a matter of good data?
Data quality in a business application sense is relatively simple to define. Typically a business unit has requirements, usually around how complete the data is and to what extent the data in it is unique (there are a wide range of additional data quality dimensions, which you can read about here). For AI and Machine Learning, however, data quality is a completely different animal. On top of the usual dimensions, the data scientist or ML engineer needs to consider if they have all the data they need to create unbiased, explainable outcomes. Put simply, if a decision has been made, then the data scientists need to be able to explain why and how this outcome was reached. This is particularly important as ML becomes part and parcel of everyday life. Turned down for credit? Chances are an algorithm has assessed a range of data sources and generated a ‘no’ decision – and if you’re the firm whose system has made that decision, you’re going to need to explain why (it’s the law!).
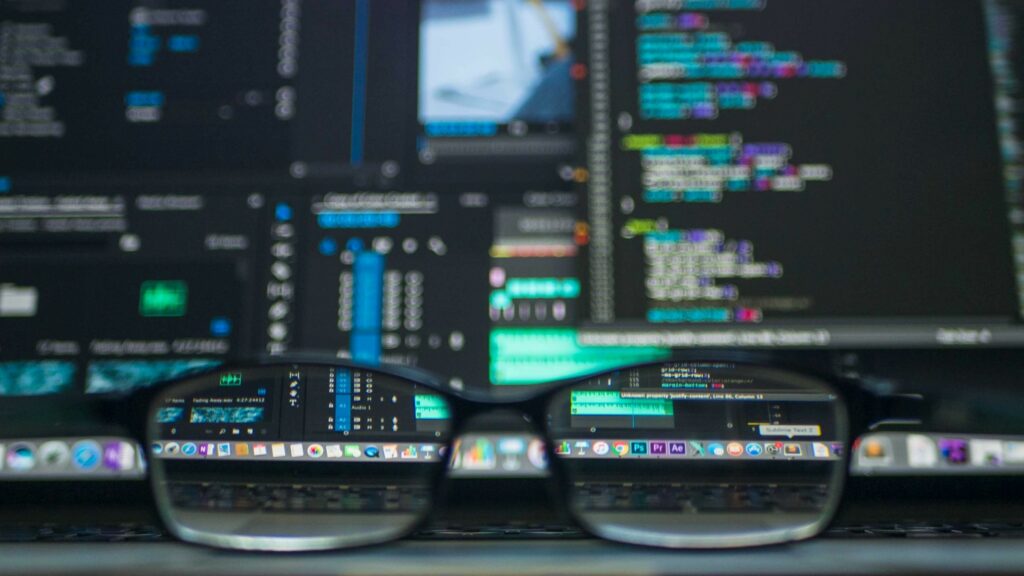
This is the point at which we return to the class in Wallace High School. The student tentatively raising their arm would have got away with it, with the model predicting patterns incorrectly, if the student had stayed silent. There was no monitoring in place to detect which user had been the ‘bad actor’ and so the flaw would have gone undetected without the student’s confession. It was, however, utterly perfect to explain the need to free algorithms from bias, for this next generation of data scientists. In the five years between now and when these students are working in industry, they will need to be fully aware of needing every possible aspect of the society people wish to inhabit being in the room when data is being classified, and models are being created.
For an industry still so populated overwhelmingly by males, it is clear that the decision to do something about what comes next lies where it always has: in the hearts, minds and hands of technology’s builders.