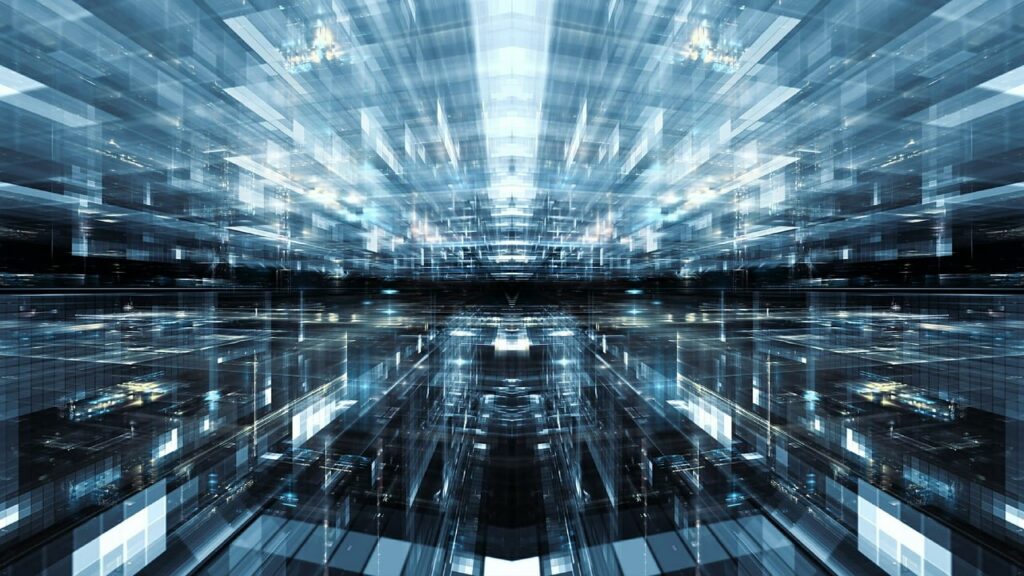
Year after year, the volume of data being generated is increasing at an unparalleled pace. For businesses, data is critical to inform business strategy, facilitate decision-making, and create opportunities for competitive advantage.
However, leveraging this data is only as good as its quality, and traditional methods for measuring and improving data quality are struggling to scale.
This is where Augmented Data Quality comes in. The term describes an approach that leverages automation to enable systems to learn from data and continually improve processes. Augmented data quality has led to the recent emergence of automated tools for monitoring and improving data quality. In this post, we’ll explain what exactly is augmented data quality, where it can be applied, and its positive impact on data management.
Why Are Traditional Approaches Struggling?
First, let’s set the scene. With an ever-growing reliance on data-driven decision-making, businesses are looking for ways to gain accurate insights, deep business intelligence, and maintain data integrity in an increasingly complex business environment.
However, measuring data quality is challenging for enterprises, due to the high volume, variety, and velocity of data. Enterprises grapple with ensuring the reliability of data that has originated from multiple sources in different formats, which can often lead to inconsistencies and duplication within the data.
The complexity of data quality management procedures, which involve data cleansing, integration, validation, and remediation, further increases the challenge. Traditionally, these have been manual tasks carried out by data stewards, and/or using a deterministic-based approach, both of which are not scalable as the volume and veracity of data grows. Now, enterprises are turning to highly automated solutions to effectively handle vast amounts of data and accelerate their data management journey and overall data management strategy.
What Is Augmented Data Quality?
Augmented Data Quality is an approach that implements advanced algorithms, machine learning (ML), and artificial intelligence (AI) to automate data quality management. The goal is to correct data, learn from this, and automatically adapt and improve its quality over time, making data assets more valuable.
Augmented data quality promotes self-service data quality management, empowering business users to execute tasks without requiring deep technical expertise. Moreover, it offers many benefits, from improved data accuracy to increased efficiency, and reduced costs, making it a valuable asset for enterprises dealing with large volumes of data.
Although AI/ML solutions can speed up routine DQ tasks, they cannot fully automate the whole process. In other words, augmented data quality does not eliminate the need for human oversight, decision-making, and intervention; instead, it complements it by leveraging human-in-the-loop technology, where human expertise is combined with advanced algorithms to ensure the highest levels of data accuracy and quality.
“Modern data quality solutions offer augmented data quality capabilities to disrupt how we solve data quality issues. This disruption – fueled by metadata, artificial intelligence/machine learning (Al/ML) and knowledge graphs – is progressing and bringing new practices through automation to simplify data quality processes.”
-Gartner®, ‘The State of Data Quality Solutions: Augment, Automate and Simplify; By Melody Chien, Ankush Jain, 15 March 2022.
GARTNER is a registered trademark and service mark of Gartner, Inc. and/or its affiliates in the U.S. and internationally and is used herein with permission. All rights reserved.
How Can Augmented Data Quality Help A Data Quality Process?
Routine data quality tasks, such as profiling and rule building, can be time-consuming and error-prone. Fortunately, the emergence of augmented data quality has revolutionized the way routine data quality tasks as performed, reducing manual effort and saving time for users. Below are some examples of where automation can add value as part of a data quality process:
Data profiling and monitoring
ML algorithms excel at recognizing patterns. For example, ML can enhance a system’s capability to manage data quality proactively, by identifying and learning patterns in data errors and corrections. Using these learnings, ML can be applied to automate routine tasks like data cleaning, validation, and deduplication.
Data Deduplication
ML can be used to identify and remove duplicate entities. Rather than simply looking for exact matches, ML algorithms such as natural language processing can identify duplicates even with minor variations, such as spelling mistakes or different formats.
Automated Validation
ML can be used to automate the data validation process. For a feature such as automated rule suggestion, the system applies ML to understand underlying data and match relevant rules to this data. The process can be further enhanced by automatically deploying suggested rules using a human-in-the-loop approach, making the process faster and more efficient.
Why Enterprises Are Embracing Augmented Data Quality
Augmented data quality is useful for any organization wanting to streamline its data quality management. Whether it’s for digital transformation or risk management, augmented data quality holds immense value. Here are a few examples of where our clients are seeing the value of augmented data quality:
Regulation and Compliance: Industries like healthcare and financial services are confronted with increasing regulatory changes. Yet, organizations often struggle to meet the demands of these regulations and must adapt quickly. By leveraging AI/ML methods to help identify data errors and ensure compliance with regulatory requirements, enterprises can efficiently minimize the potential risks associated with poor data quality.
Use Cases: Single Customer View, Sanctions matching.
Business analytics: With complete, and consistent data, organizations can leverage analytics to generate accurate insights and gain a competitive edge in the market. Through AI/ML, data quality processes can be automated to quickly produce analytics and predict future trends within the data.
Use Cases: Data preparation & Enrichment, Data & Analytics Governance.
Modern Data Strategy: Data quality is a foundational component of any modern data strategy, as data sources and business use cases expand. By leveraging augmented data quality within a modern data strategy, organizations can experience greater automation of manual processes, such as rule building and data profiling.
Use Cases: Data Quality Monitoring & Remediation, Data Observability
Digital Transformation: Enterprise-wide digital transformation is taking place across all industries to generate more value from data assets. Automation plays a crucial role in enabling scalability, reducing costs, and optimizing efficiencies.
Use Cases: Data Harmonization, Data Quality Firewall
Adopting augmented data quality within an organization represents a transformative step towards establishing a data-driven culture, where data becomes a trusted asset that drives innovation, growth, and success. The automation of process workflows reduces dependence on manual intervention, saving time and resources while enhancing efficiency and productivity. Moreover, augmented data quality increases accuracy, reliability, and compliance, enhancing customer experiences and improving an organization’s competitive advantage.
In conclusion, the seamless integration of augmented data quality within essential business areas offers significant benefits to organizations seeking to maximize the value of their data.
Find out more about Datactics Augmented Data Quality platform in the latest news from A-Team Data Management Insight.