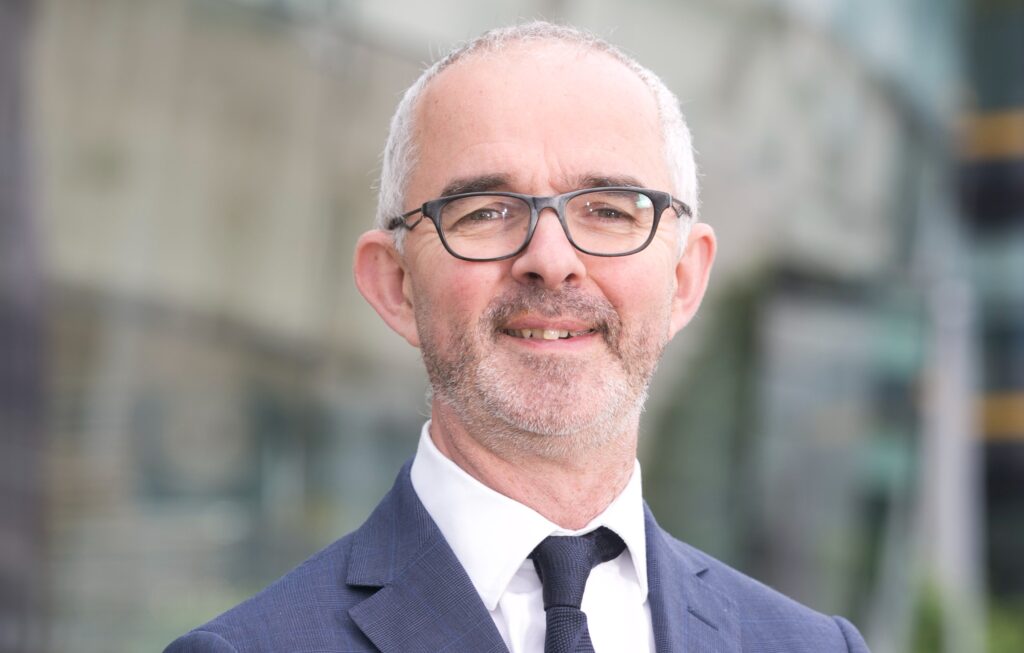
Amidst ever-changing regulatory requirements and hype around the potential of data-driven technologies, demand for better quality data in the financial industry has never been higher. Stuart Harvey, CEO of Datactics, writes that a self-service approach could be the key that unlocks significant competitive advantage for financial firms.
Demand for higher data quality in the financial industry has exploded in recent years. A tsunami of regulations such as BCB23, MiFID and FATCA stipulate exacting standards for data and data processes, causing headaches for compliance teams.
At the same time, financial firms are trying to grasp the fruitful benefits of becoming more data and analytics driven. They are embracing technologies such as artificial intelligence (AI) and machine learning (ML) to get ahead of their competitors.
Through their attempts at meeting regulatory requirements and gaining meaningful insights from these technologies, they are coming to the realisation that high quality, reliable data is absolutely critical – and extremely difficult to achieve.
But there is an evolution underway. At the core of this evolution is the establishment of ‘self-service’ data quality whereby data owners have ready access to robust tools and processes to measure and maintain data quality themselves, in accordance with data governance policies. This not only simplifies the measuring and maintenance of data quality; it can help turn it into a competitive advantage.
High quality data in demand
As the urgency for regulatory compliance and competitive advantage escalates, so too does the urgency for high data quality. But it’s not plain sailing and there is a variety of disciplines required to measure, enrich, and fix data.
Legacy data quality tools were traditionally owned by IT teams as by its very nature, digital data can require significant technical skills to manipulate. However, this created a bottleneck as maintaining data also requires significant knowledge about its content – what good data and bad data looks like, and what its context is – and this resides with those who use the data, rather than a central IT function.
Each data set will have its own users within a business who have the special domain knowledge required to maintain the data. If a central IT department is to maintain quality of data correctly, it must liaise with many of these business users to correctly implement the controls and remediation required. This creates a huge drain on IT resources and a slow-moving backlog of data quality change requirements within IT that simply can’t keep up.
Due to the lack of scalability of this method, many have come to the realisation that this isn’t the answer and so have started moving data quality operations away from central IT back into the hands of business users.
This move can accelerate information measurement, improvement and onboarding processes, but it isn’t without flaws. It can be difficult and expensive for business users to meet the technical challenges of this task and unless there is common governance around data quality there is the risk of a ‘wild west’ scenario where every department manages data quality differently across the business.
Utilising self-service data quality platforms
Financial firms are maturing their data governance processes and shifting responsibility away from IT to centralised data management functions. These Chief Data Officer functions are seeking to centralise data quality controls while empowering data stewards who know and understand the business context of the data best.
As part of this shift, they require tooling that matches the skills and capabilities of different profiles of user at each stage of the data quality process. And this is where self-service data quality platforms come into a league of their own. However, not all are made equal and there a few key attributes to look for.
For analysts and engineers, a self-service data quality platform needs to be able to provide a profiling and rules studio that enables the rapid profiling of data and configuring/editing of rules in a GUI. It must also offer a connectivity and automation GUI to enable DataOps to automate the process.
For business users, it needs to offer easy-to-understand visualisation or dashboard so they can view the quality of data within their domain, and an interface so they can remediate records which have failed a rule or check.
Agility is key to quickly onboard new datasets and the changing data quality demands of end consumers such as AI and ML algorithms.
It should be flexible and open, so it integrates easily with existing data infrastructure investment without requiring changes to architecture or strategy and advanced enough to make pragmatic use of AI and machine learning to minimise manual intervention.
This goes way beyond the scope of most stand-alone data prep tools and ‘home grown’ solutions that are often used as a tactical one-off measure for a particular data problem.
Gaining an edge
The move towards a self-service oriented model for data quality is a logical way to keep up with the expanding volumes and varieties of information being discovered, accessed, stored or made available. However, data platforms need to be architected carefully to support the self-service model in accordance with data governance to avoid the ‘wild west’ scenario.
Organisations that successfully embrace and implement such a platform are more likely to benefit from actionable data, resulting in deeper insight and in more efficient compliance, in turn unlocking significant competitive advantage.
And for more from Datactics, find us on Linkedin, Twitter or Facebook.